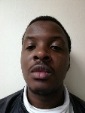
Antonius Mamphiswana
University of the Witwatersrand, South Africa
Title: Terrain classification for unmanned ground vehicle in an unstructured environment
Biography
Biography: Antonius Mamphiswana
Abstract
Identifying the travesability of a terrain for unmanned ground vehicle (UGV) is of great importance. This is because UGV are intended to operate in a dynamic environment where there are many obstacles (dynamic and static obstacle). For the UGV to be able to identify the travesability of the scene, the UGV needs to be continuously sensing and predicting the travesability of the terrain around it. To do the sensing, we used 5 color cameras and a velodyne lidar. To predict if the terrain is traversable or not we have used a deep convolutional neural network (DCNN) and height difference was calculated from point cloud data. The DCNN will classify RGB images and height difference approach will classify point cloud data from the velodyne lidar. The height difference approach was used by Stanley the car that won the DARPA grand challenge 2005. The results from both the classifier (DCNN) and the height difference have time stamp which will help in fusing the results. Both the approaches produce good results in identifying the travesability of the terrain.