Day 1 :
Keynote Forum
Juan Pedro Bandera Rubio
University of Malaga, Spain
Keynote: Why socially assistive robots?
Time : 9:15 - 10:00
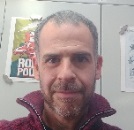
Biography:
Abstract:
Keynote Forum
Yuji Iwahori
Chubu University, Japan
Keynote: Application of computer vision to endoscope and SEM images using neural network learning
Time : 10:00-10:45
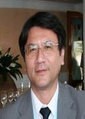
Biography:
Yuji Iwahori has completed his BSc degree from Department of Computer Science, Nagoya Institute of Technology, MS and PhD degree from the Department of Electrical and Electronics, Tokyo Institute of Technology in 1985 and 1988, respectively. He had joined Nagoya Institute of Technology in 1988 and then became a Professor in 2002. He has joined Chubu University as a Professor since 2004 with experience of the Department Head of Computer Science. In the meanwhile, he has been a Visiting Researcher of the University of British Columbia Computer Science, Canada. He has also been a Research Collaborator with Indian Institute of Technology, Guwahati. His research interests include computer vision and application of machine learning. He has published over 220 scientific papers of journals and international conferences.
Abstract:
In the computer vision and machine learning fields, image recognition and its application technologies are more and more becoming popular in recent years. In this talk, application of computer vision to medical endoscope and SEM images using neural network is introduced for the approaches we have developed in recent years. Endoscope images are used for the supporting system of the medical diagnosis including 3D shape recovery and pattern classifications, where automatic polyp detection and classification of benign or malignant are investigated based on the recent machine learning approaches including deep learning, while SEM images are used to recover 3D shape for the industrial applications using neural network.
Keynote Forum
Adrian Fazekas
RWTH Aachen University , Germany
Keynote: Applications of microscopic traffic data analysis in intelligent transport systems
Time : 11:00-11:45
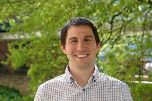
Biography:
Abstract:
Keynote Forum
Emdad Khan
CEO, InternetSpeech, USA
Keynote: Natural Language Based Intelligent Robot to Advance Industrial Automation and Digital Manufacturing
Time : 11:45-12:30
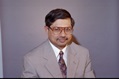
Biography:
Emdad Khan is Chairman of InternetSpeech which he founded with the vision to develop innovative technology for accessing information on the internet anytime, anywhere, using just an ordinary telephone and the human voice. He is a Faculty at Maharishi University of Management, Iowa, USA and a Research Professor at Southern University, Louisiana, USA. He holds 23 patents and published over 75 journal and conference papers on intelligent internet, natural language processing/understanding, machine learning, big data, bioinformatics, software engineering, neural nets, fuzzy logic, intelligent systems and more. He has developed the prototype of voice internet and semantic engine using brain-like approach.
Abstract:
Automation had started in the late 18th century with mechanization of textile industry and initiated the first industrial revolution. It then continued and started the second industrial revolution in early 20th century when Henry Ford mastered the moving assembly line and ushered in the age of mass production. The first two industrial revolutions made people richer and more urban. The biggest benefit of automation is that it saves labor. However, it is also used to save energy and materials and to improve quality, accuracy and precision. Now a third revolution is under way. Manufacturing is going digital. And what would be the next? We believe it will be intelligent agent based robots (soft-bots) that will take industrial automation digital manufacturing to the next level. Such robots will also communicate more naturally with human and machine. The dominant mechanism for natural communication is Natural Language Understanding (NLU) and processing. This study focuses on the key issues of robots to drive industrial/manufacturing automation and discusses specifically the NLP (Natural Language Processing) algorithms and Intelligent Agent (IA); the two core components of future automation. NLP is very important for the best HCI (Human Computer Interaction): Natural language based interaction, in general, is the most preferred communication with man as well as machine. Clearly understanding user’s input by IA is also the key to take necessary actions. And NLP can also make the search space significantly smaller in taking necessary actions. The core to NLP is a semantic engine that can understand the semantics and is critical for any complex NLP based applications. Semantic Engine is also the key for cognitive computing. We will discuss a Semantic Engine using Brain-Like Approach (SEBLA) and associated NLP & NLU to address the key problems of intelligent robot based automation. SEBLA based NLU (SEBLA-NLU) resembles human Brain-Like and Brain-Inspired algorithms and hence is good at dealing with natural language based interactions. In fact, SEBLA and IA are also very critical to solve most Big Data problems, especially when data is dominated by text. Our proposed SEBLA and IA based solution would make it much easier to effectively use robots (and softbots) by non-technical, semiliterate, illiterate as well as by technical people.
Keynote Forum
Zhou XING
Borgward Automotive Group, USA
Keynote: Predictions of short-term driving intention using recurrent neural network on sequential data
Time : 13:30-14:15

Biography:
Zhou Xing has completed his PhD degree in Particle Physics with his thesis focusing on large scale statistical data analysis, utilizing neural network methodologies on various analysis projects at LHCb experiment at CERN. He has joined Stanford Linear Accelerator Center (SLAC) National Laboratory as Engineering Physicist/Faculty and Staff, working on data acquisition and analysis. He has also joined a leading China EV company, NIO, where he specializes on a few deep-learning driven fields related to applications of autonomous driving, including supervised learning for semantic segmentations/road segmentations, moving object detections, trajectory prediction using optical camera as well as LIDAR measurements, reinforcement learning of continuous control, policy-based semi-model controlled reinforcement learning methods, etc.
Abstract:
A prediction of driver’s intentions and their behaviors using the road is of great importance for planning and decision making processes of autonomous driving vehicles. In particular, relatively short-term driving intentions are the fundamental units that constitute more sophisticated driving goals, behaviors, such as overtaking the slow vehicle in front, exit or merge onto a high way, etc. While it is not uncommon that most of the time human driver can rationalize, in advance, various on-road behaviors, intentions, as well as the associated risks, aggressiveness, reciprocity characteristics, etc., such reasoning skills can be challenging and difficult for an autonomous driving system to learn. In this article, we present a disciplined methodology to build and train a predictive drive system, which includes various components such as traffic data, traffic scene generator, simulation and experimentation platform, supervised learning framework for sequential data using Recurrent Neural Network (RNN) approach, validation of the modeling using both quantitative and qualitative methods, etc. In particular, the simulation environment in which we can parameterize and configure relatively challenging traffic scenes customize different vehicle physics and control for various types of vehicles such as cars, SUV, trucks, test and utilize high definition map of the road model in algorithms, generate sensor data out of Light Detection and Ranging (LIDAR), optical wavelength cameras for training deep neural networks is crucial for driving intention, behavior, collision risk modeling since collecting statistically significant amount of such data as well as experimentation processes in the real world can be extremely time and resource consuming.
Keynote Forum
Sunan Huang
Temasek Laboratories-National University of Singapore, Singapore
Keynote: Distributed collision avoidance control for multi-unmanned aerial vehicles
Time : 14:15-15:00
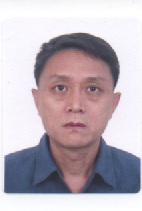
Biography:
Sunan Huang has completed his PhD degree from Shanghai Jiao Tong University. He was a Postdoctoral Fellow in the Department of Electrical Engineering and Computer Sciences, University of California at Berkeley. He was also a Research Fellow in the Department of Electrical and Computer Engineering, National University of Singapore, a Visiting Professor in Hangzhou Dianzi University. He is currently a Senior Research Scientist in Temasek Laboratories, National University of Singapore. He has co-authored several patents, more than 120 journal papers and four books entitled Precision Motion Control, Modeling and Control of Precise Actuators, Applied Predictive Control and Neural Network Control: Theory and Applications. He is also a Member of the Editorial Advisory Board, Journal of Recent Patents on Engineering, a Reviewer Editor of Journal of Frontiers in Robotics and AI and an Associate Editor of The Open Electrical and Electronic Engineering Journal.
Abstract:
Currently, cooperative control of a multiple Unmanned Aerial Vehicle (UAV) system is attracting growing interest. This is motivated by growing number of everyday civil and commercial UAV applications. One of the core problems in the multi UAV system is motion planning, where each UAV navigates path to the target by sharing other UAV information. This requires collision-free path during the UAV motion control. Thus, the topic of UAV collision avoidance has driven a development of various control technologies in this area. In this talk, we first review the development of multiple UAV systems and collision avoidance. Therefore, we focus on a distributed collision avoidance algorithm which is proposed in a multi-UAV system. The basic idea is to use the cooperative control concept to generate heartbeat message, where multi-UAV communication is used to exchange UAV information and the fusion technology is used to merge them. With the heartbeat message fused, the own UAV is to select the velocity command to avoid only those UAVs or obstacles which are within a certain range around the own UAV. The velocity obstacle algorithm is adopted for collision avoidance control. This control is in a distributed form and each UAV independently makes its own decision. Finally, in this talk, we will show the flight test of the proposed method implemented on several real UAVs.
Keynote Forum
Boian Mitov
CEO
Keynote: STEM Robotics Made Easy: An easy way for students to program robots
Time : 10:15 -11:00
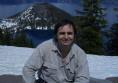
Biography:
He has over 30 years of overall programming experience in large variety of software problems, and is a regular contributor to the Blaise Pascal Magazine http://www.blaisepascal.eu .
Abstract:
Keynote Forum
Zhou Xing
Directory of Artificial Intelligence for Autonomous Driving Borgward Automotive Group
Keynote: Predictions of short-term driving intention using recurrent neural network on sequential data
Time : 13:30-14:15

Biography:
Abstract:
Keynote Forum
Yuji Iwahori
Chubu University JAPAN
Keynote: Application of Computer Vision to Endoscope and SEM Images Using Neural Network Learning
Time : 10:00-10:45

Biography:
Abstract:
- Special Session
Location: Polaris II
Session Introduction
Dr. Jun Kurihara
The Canon Institute for Global Studies, Japan
Title: The significance of robot safety standards for the development of life support robots
Time : 15:15-16:15
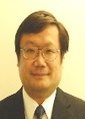
Biography:
Jun Kurihara is a Research Director of The Canon Institute for Global Studies (CIGS), a Tokyo-based think tank (2009 to till date). He also serves as a Corporate Director of a Japanese company (Ono Pharmaceutical, 2013 to till date) and teaches as a Visiting Professor at Kwansei Gakuin University located in Hyogo Prefecture, Japan (2006 to till date). Between 2003 and 2012, he was a Senior Fellow at the Harvard Kennedy School, Ash Center and the Center of Business and Government (CBG). Currently he is working on Japanese companies’ innovation strategies including service robotics and artificial intelligence. He has served as a Chairman and Member of various committees established by the government and business organizations including a Senior Member of the Japan Statistics Council for the Government of Japan. He has earned an MS from the Graduate School Agriculture of Kyoto University.
Abstract:
Japan is the forerunner in the world in terms of aging population. Under these circumstances, life support robots are promising tools to lead to a higher Quality Of Life (QOL) for the elderly along with physically challenged people; both young and old. However, there has been a jumble of ideas and prototypes, leading to a cul-de-sac with respect to the future development of caregiver and self-reliance support robots. This presentation proposes a promising approach to this cul-de-sac. This impasse has been caused by three factors. First, robots are extremely expensive, unaffordable for caregivers and patients. Second, robot markets are extremely compartmentalized and isolated. Third, the primary cause of compartmentalized market has been a confusing patchwork of standards. Thus far, the cul-de-sac has brought about a lackadaisical growth of life support robots, compared with the case of industrial robots that are experiencing a galloping growth, especially in East Asia. Accordingly, the time has come to draw up a new road map toward a higher speed of robot diffusion. The most effective approach would be the establishment of globally encompassing and trustworthy safety standards. They could provide a firm foundation for a globalized and integrated market of life support robots. Individual markets, thanks to universally applicable safety standards, would be loosely interconnected; those markets would include not only the market for elderly care, but also medical, educational, business and military services as well as industrial robots, leading to a larger pool of components and related technologies. The resulting larger pool could reduce the prices of life support robots and accelerate their diffusion. The establishment of globally encompassing safety standards requires an institutional framework which could play a leadership role. Japan has a robot safety center (RSC); only one in the world to systematically propose safety standards and guidelines for life support robots.
- Poster Presentations
Location: Polaris II
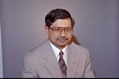
Chair
Emdad Khan
Internet Speech, USA
Session Introduction
Baoming Zhang
Zhengzhou University, China
Title: Deep feature learning for unsupervised change detection in high-resolution multi-temporal and multi-source images
Time : 16:15-16:35
Biography:
Baoming Zhang is currently a full Professor at Department of Photogrammetry and Remote Sensing, Zhengzhou Institute of Surveying and Mapping. His research interests are in the areas of digital photogrammetry, remote sensing, image processing and pattern recognition.
Abstract:
Multi-temporal imagery change detection is growing in popularity among many applications, such as geography information updating, disaster monitoring, agriculture monitoring. With the improvement of spatial resolution, more subtle change information is expected to be detected. However, high-resolution imaging systems usually have low temporal resolution, resulting that multi-source images have to be considered to satisfy different kinds of applications, which brings increased challenges for change detection. Recently, deep learning is a fast-developing domain, making it possible for unsupervised abstract feature extraction of remote sensing images. For this reason, this paper proposed an unsupervised change detection approach using deep feature learning for high-resolution multi-temporal images acquired by different sensors. First, to obtain initial and reliable change information from bi-temporal images, multiple features are extracted including spectral features, texture features and edge features. Through utilizing these features jointly, specific rules are designed to select robust changed and unchanged samples automatically. Then, the bi-temporal multi-source images are layered as original change feature and Stacked De-noising Auto-Encoder (SDAE) is introduced for transforming the feature into a new feature space, where change information is represented deeply. Finally, the change detection model is constructed by adding a supervised classifier to the deeply learned features and the change information can be obtained by feeding the samples into the model with fine-tuning. Experiments with multi-temporal images from different sources demonstrate the effectiveness and robustness of the proposed approach.
Zhihui Gong
Zhengzhou University, China
Title: Joint salient feature and convolutional neural network for ship detection in remote sensing images
Time : 16:35-16:55
Biography:
Zhihui Gong is currently a full Professor at Department of Photogrammetry and Remote Sensing, Zhengzhou Institute of Surveying and Mapping. His main research interests include digital photogrammetry, remote sensing and image processing.
Abstract:
Due to the interference of clouds, sea waves, islands and other uncertain conditions on sea surface in satellite images, the majority of ship detection algorithms show poor performance in object detection and recognition. This paper proposes a method based on joint visual salient feature and convolutional neural network. First, the saliency map of image can be calculated by Phase spectrum of Fourier Transform (PFT), which is based on analysis of frequency domain. PFT can effectively suppress the interference of clouds and sea waves, but the distinction between background and ship is not notable. To solve this problem, adaptive logarithmic transformation is used to enhance the saliency map. Then, the gray morphological operation is adapted to eliminate noise areas and fill small holes. An adaptive image segmentation algorithm is used to extract all the salient area as the candidates. Finally, with a small number of ship samples and the idea of transferring learning to a simple convolutional neural network model can be trained. All candidate areas will be predicted by the model and the ships will be exactly detected and recognized. The experiments results show that our algorithm can effectively eliminate the interference of various factors such as cloud and islands and has the advantage on dealing with various kinds and scales of ships.
- Speaker Session: Robotics | Artificial Intelligence | Medical Robotics | Industrial Robot Automation | Humanoid Robots | Human-Robot Interaction | Deep Learning
Location: Polaris II
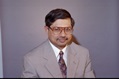
Chair
Emdad Khan
Internet Speech, USA
Session Introduction
Rattapon Thuangtong
Mahidol University, Thailand
Title: Robotic hair transplantation
Time : 13:45-14:15
Biography:
Rattapon Thuangtong is 2nd year PhD student in Biomedical Engineering, Faculty of Engineering, Mahidol University, Bangkok, Thailand and he is expertise in Hair transplantation-Robotic, FUT, FUE, Synthetic hair transplantation and he is professional doctor from Siriraj Hospital, Thailand
Abstract:
Follicular unit transplantation was classified into two techniques: (1) Strip harvesting follicular unit transplantation and (2) Follicular Unit Extraction (FUE). Artas robotic hair transplantation is the newly developed machine that use robotic arm to operate FUE. By using skin tensioner and photo sensor, robot machine can perceive the direction of each hair follicle within that frame and can process the robotic arm with two sets of punch (sharp punch and dull punch) perform FUE precisely. Artas robotic hair transplantation can improve FUE by increasing the speed and accuracy of harvesting. After using Artas robotic hair transplantation, for a few years, Artas function very well within the central area of the occiput that we called sweet spot. We found that there are some limitations of Artas robotic hair transplantation such as: (1) Limitation in the temporoparietal area of the scalp, (2) Limitation for the lower occipital area of the scalp (3) Limitation for female and senile patient, and (4) Artas has some limitation for previous surgery patients. We try to improve the result by: (1) Selecting the proper patient, not too old because of fragile tissue, (2) Perform tumescent solution injection as much as possible to decrease the would size and decrease the transection, (3) Upgrade the hardware and software of the Artas into 3,000 RPM version, (4) Combine using handheld motorized FUE machine to perform FUE in the temporoparietal and lower occipital, (5) Extract the hair follicles during on skin tensioner, and (6) Using team working to maximize the speed. Finally, robotic hair transplantation helps surgeon to perform FUE quicker and more accuracy. It is the important milestone on hair transplantation, but it needs more improvement for the best outcome of the patients.
Martin Heide Jørgensen
The Maersk Mc-Kinney Moller Institute-University of Southern Denmark, Denmark
Title: Industry 4.0, robotics and automation in the production environment: Future trends and challenges in product design
Time : 14:15-14:45
Biography:
Martin Heide Jørgensen is a Program Coordinator I4.0 since 2017 at University of Southern Denmark, The Maersk Mc-Kinney Moller Institute. He had completed his PhD from Aalborg University in the area of Fracture Mechanics. Later, he has contested different jobs, all in the area of public research and education, including a period of 7 years as Head of Department at Aalborg University. Within the last 3-4 years, his research area has been digitalization and I4.0.
Abstract:
Together with the digitalization, the frames for product design are changing substantial. Tools for simulation and digital twin representations now can be connected directly with the CAD systems. This means that the optimization of the product or the mechanical solution now can be handled in a multi objective system involving performance, reliability, production technologies and value chain analysis. A very important feature is the possibility of including systematic performance studies of the use of the product or mechanical solution. This can be done still by empirically means, but due to the digitalization, also using systematic sensor input. This enables the possibility of a more user-oriented perspective in the design, but also that the design can be defined in a more open manner, where the customer by digital tools can define some free elements and functionalities within a given frame of design freedom. To realize these new possibilities a more flexible and agile production system is required. In this sense the number of robot, flexible production units and new digitalized technologies are introduced in the production environments. This leads off cause to a higher degree of flexibility, but also a need for planning and control to obtain an economic feasible productivity. For many industrial CEO’s there is a big challenge in finding the right strategy and track in the world of digitalization to balance the cost and productivity with the ability to act agile and in harmony when new marked possibilities occur. The business strategy and strategy for optimizing of products and production setup is getting more complex and specific for the individual company. The challenge is to find some generic tools or methods to support this development.
Aleksei Yuzhakov
Promobot LLC, Russia
Title: Promobot: Autonomous service robot for business
Time : 14:45-15:15
Biography:
Alexey Yuzhakov has completed his PhD at the Pern National Research University. He is the CEO and Chairman of the board of directors of Promobot, a service robotics company.
Abstract:
Promobot is an autonomous service robot for your business. It is designed to work in crowded spaces, where, by moving autonomously, it helps people with navigation, communicates and answers any questions, shows promotional materials and remembers everyone with whom it interacted. Promobot attracts the maximum audience to the company’s products, as well as takes the people out of the process since it works autonomously. Today, several hundred of Promobot robots work in 26 countries, almost on every continent. They work as administrators, promoters, hosts, museum guides in such companies as NPF Sberbank, Beeline, Museum of Contemporary History of Russia, the Moscow Metro, and are able to increase financial performance of companies, quality of service and customer loyalty. Combination of the technologies of speech recognition, face recognition, speech synthesis, integrated linguistic database and ability to look up the information on the Internet allowed us to form a unique experience for the customers. When they come up to Promobot they see not only a voice assistant, ATM machine, toy or a terminal, they see a robot and they have to communicate with it like with a robot. And the robot has to communicate with them, answering their questions, helping them, showing them the way, giving them a pass, etc. Promobot is not a part of something fancy which you only see on Youtube, it is a part of our life and it is time to face it.
- Workshop
Location: Polaris II
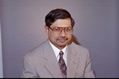
Chair
Emdad Khan
Internet Speech, USA
Session Introduction
Caner Sahin
Imperial College London, UK
Title: Category-level 6D object pose recovery in depth images
Time : 15:15-16:00
Biography:
Caner Sahin is a PhD student in Imperial Computer Vision and Learning Lab at the Department of Electrical and Electronic Engineering of Imperial College, London. His PhD research is based on computer vision and machine learning. Particularly, he is working on object recognition, detection and 6D pose estimation.
Abstract:
Intra-class variations, distribution shifts among source and target domains are the major challenges of category level tasks. In this study, we address category level full 6D object pose estimation in the context of depth modality, introducing a novel part-based architecture that can tackle the above mentioned challenges. Our architecture particularly adapts the distribution shifts arising from shape discrepancies and naturally removes the variations of texture, illumination, pose, etc. so we call it as Intrinsic Structure Adaptor (ISA). We engineer ISA based on the innovations: (1) Semantically Selected Centers (SSC) are proposed in order to define the 6D pose at the level of categories, (2) 3D skeleton structures, which we derive as shape-invariant features are used to represent the parts extracted from the instances of given categories and privileged one-class learning is employed based on these parts, (3) graph matching is performed during training in such a way that the adaptation/generalization capability of the proposed architecture is improved across unseen instances. Experiments validate the promising performance of the proposed architecture.
Biography:
Suranjana Trivedy is currently a Faculty in GATE IIT Training Institute, India. She was Research Scholar in IIIT Hyderabad. Her current research interests are in the area of artificial intelligence, building intelligent system, robotics, autonomous vehicle and UAV.
Abstract:
The aim of the project is to build an intelligent naturally dialog-able machine, which can talk with the depressed and lonely older people and can finally become their true companion. Specifically, the research goal was to build an automatic system to understand multimodal emotion, including facial expressions, speech tone and the linguistic emotions. For that purpose we used semi-supervised learning methods, standard Natural Language Processing (NLP) (uni-gram) and Information Retrieval (IR) Term-Frequency Inverse-Document-Frequency (TF-IDF) techniques to analyze emotion from text. We have extracted emotion from facial images. Overall aim is to create intelligent companion bot.